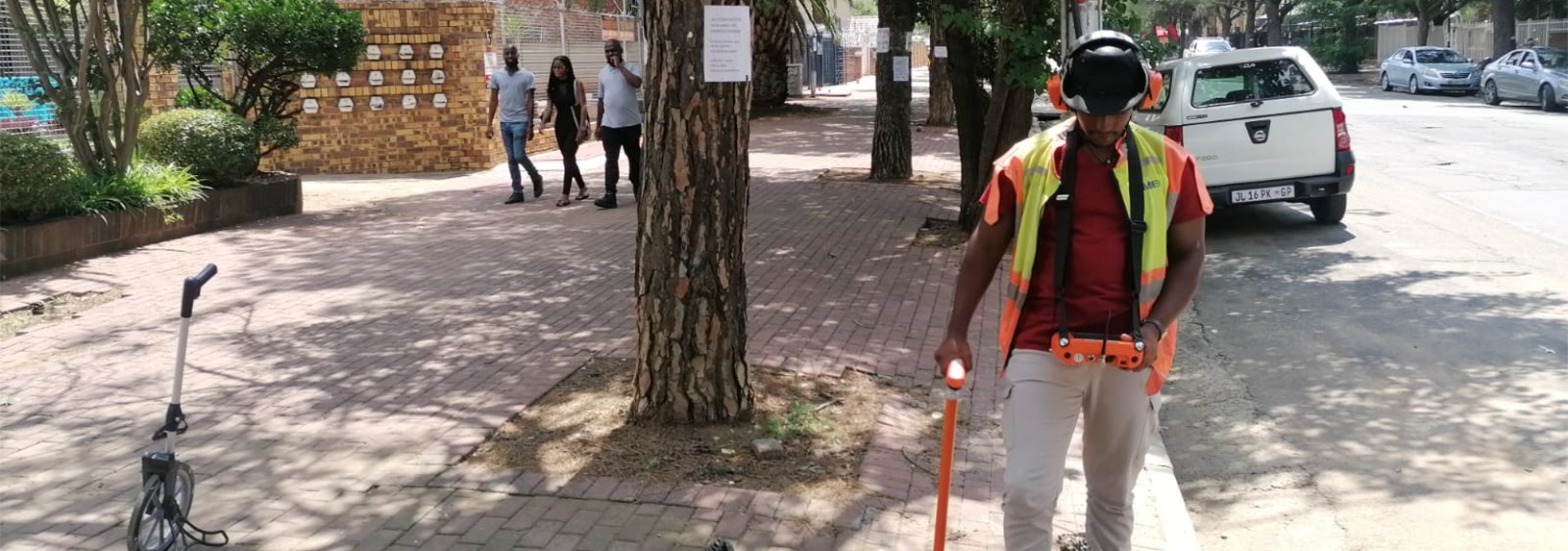
Figure 1 displays an example of the results from the preliminary model, with the output generated through the following process:
- The in-office user selects the CCTV file or folder directory of multiple files and initiates the model.
- The model then runs without human supervision through each video frame and searches for any irregularities learned during the training stage.
- If the model identifies an object (irregularity), the model outputs the following data subject to a threshold confidence level:
- If the confidence of the detection is high, the model outputs the data shown in Table 1. In addition to this output, the model can retrieve any metadata from the video file, as requested by the client. For example, if the drone is GPS enabled, then the X and Y GPS coordinates of the identified irregularity can be extracted.
- If the confidence of the detection is low, the irregularity is identified as an edge case and the model trims the video for later inspection by the in-office technician.
- The model then pushes the extracted data to a database, or the like, for record keeping.
- Once the model is complete, the in-office technician needs only review the CCTV footage of objects identified by the model with low confidence (edge cases), thereby substantially reducing the time required for the review. As the model is used, the reviewed low-confidence detections are used by the SMEC AI team to improve the accuracy of the model.
- Thereafter, the Project Engineer can assess the structured data to make data-driven decisions on the pipe replacement or point repair requirements.




Transmission Systems of the future need to rapidly adapt to the new world of sustainable energy. Grids need to expand to accommodate the growing new sources of clean energy and adjust to the variability and intermittency nature of such sources.

Transport mega-projects are reshaping the future of urban mobility, driving innovations that enhance connectivity, support communities, and enable economic growth and sustainability. Through cutting-edge technology and large-scale infrastructure, these projects are transforming cities by reducing congestion, improving environmental outcomes and facilitating seamless travel.

Transportation geotechnics forms the backbone of global infrastructure, supporting roads, railways, ports, and airports that drive economic growth and societal progress. As urbanisation accelerates and economies expand, the geotechnical challenges associated with constructing and maintaining resilient infrastructure have grown increasingly complex. The field must not only address capacity needs but also align with sustainability and resilience goals, making it more crucial than ever to innovate and adapt.

Across the vast and diverse landscapes of rural Africa, many communities face significant barriers to accessing essential infrastructure. Limited access to clean water, sanitation, reliable transportation, and consistent energy supply impedes daily life, and deepens existing inequalities.